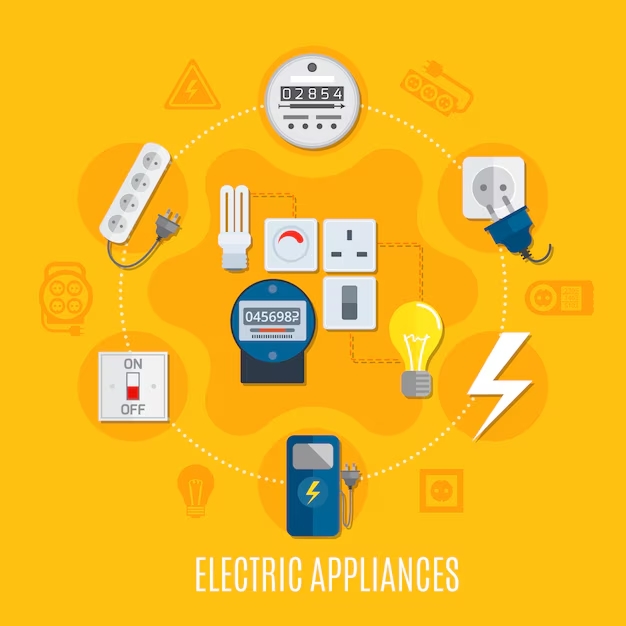
In the modern era, electricity demand is crucial to support various human activities, especially in densely populated areas such as Java-Bali. Daily fluctuations in electricity consumption directly impact the revenue of electricity companies. To anticipate future economic changes and avoid losses, accurate forecasting of electricity consumption revenue is needed. This article discusses the use of Jordan Recurrent Neural Networks (JRNN) to forecast short-term electricity consumption revenue in the Java-Bali electricity system.
Methodology
This study uses secondary data on electricity consumption from the 500 kV electricity system in the Java-Bali region, collected from January to March 2012. Data were collected every half hour and grouped by day of the week. Each group was then multiplied by the 2012 Basic Electricity Rate (TDL) of Rp. 1010.00 to obtain the electricity consumption revenue.
The JRNN model was trained using electricity consumption data and compared with a traditional Artificial Neural Network (ANN) model. Evaluation was conducted using Sum of Square Error (SSE) and Mean Square Error (MSE).
Results
The forecasting results show that both JRNN and ANN produced consistent patterns for weekdays but were inaccurate for weekends. This indicates that electricity consumption on weekends has different characteristics from weekdays. Lower SSE and MSE values in JRNN compared to ANN indicate that JRNN is more accurate in forecasting electricity consumption revenue in the Java-Bali system.
Discussion
Electricity consumption in Indonesia shows different patterns between weekdays and weekends. On weekdays, electricity consumption is more stable due to fixed working hours. Conversely, on weekends, human activities are more varied, leading to irregular electricity consumption. Although JRNN produced higher error values on weekends, the model still performed better than traditional ANN.
Conclusion
Short-term electricity consumption revenue forecasting using JRNN shows more accurate and faster results compared to traditional ANN. Electricity consumption in the Java-Bali electricity system is more consistent on weekdays than on weekends. To improve accuracy, it is recommended to train the JRNN model with different parameters and a broader dataset for weekends.
Source Journal : https://ejournal.unair.ac.id/JISEBI/article/download/9027/5663